PRIMES Response to COVID-19
9) Catherine Li and Daniel Lazarev (MIT), Spatiotemporal risk prediction for infectious disease spread and mortality (28 Nov 2023; arXiv.org, 5 Dec 2023)
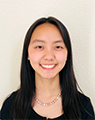
With the outbreak of the COVID-19 pandemic, various studies have focused on predicting the trajectory and risk factors of the virus and its variants. Building on previous work that addressed this problem using genetic and epidemiological data, we introduce a method, Geo Score, that also incorporates geographic, socioeconomic, and demographic data to estimate infection and mortality risk by region and time. We employ gradient descent to find the optimal weights of the factors’ significance in determining risk. Such spatiotemporal risk prediction is important for informed public health decision-making so that individuals are aware of the risks of travel during an epidemic or pandemic, and, perhaps more importantly, so that policymakers know how to triage limited resources during a crisis. We apply our method to New York City COVID-19 data from 2020, predicting ZIP code-level COVID-19 risk for 2021.
8) Paula Bergero, Laura P. Schaposnik, and Grace Wang (PRIMES), Correlations Between COVID-19 and Dengue (arXiv.org, 27 Jul 2022), published in Nature Scientific Reports (27 Jan 2023)
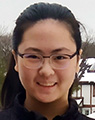
A dramatic increase in the number of outbreaks of Dengue has recently been reported, and climate change is likely to extend the geographical spread of the disease. In this context, this paper shows how a neural network approach can incorporate Dengue and COVID-19 data as well as external factors (such as social behaviour or climate variables), to develop predictive models that could improve our knowledge and provide useful tools for health policy makers. Through the use of neural networks with different social and natural parameters, in this paper we define a Correlation Model through which we show that the number of cases of COVID-19 and Dengue have very similar trends. We then illustrate the relevance of our model by extending it to a Long short-term memory model (LSTM) that incorporates both diseases, and using this to estimate Dengue infections via COVID-19 data in countries that lack sufficient Dengue data.
7) Varun Suraj (PRIMES), Catherine Del Vecchio Fitz, Laura Kleiman, Suresh Bhavnani, Chinmay Jani, Surbhi Shah, Rana McKay, Jeremy Warner, and Gil Alterovitz, SMART COVID Navigator, a Clinical Decision Support Tool for COVID-19 Treatment: Design and Development Study , published in Journal of Medical Internet Research 24, no. 2 (18 Feb 2022)
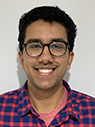
COVID-19 caused by SARS-CoV-2 has infected 219 million individuals at the time of writing of this paper. A large volume of research findings from observational studies about disease interactions with COVID-19 is being produced almost daily, making it difficult for physicians to keep track of the latest information on COVID-19’s effect on patients with certain pre-existing conditions.
6) Daniel Xu, Modeling of Network Based Digital Contact Tracing and Testing Strategies for the COVID-19 Pandemic (26 Dec 2020; arXiv.org, 28 Dec 2020), published in Mathematical Biosciences , vol. 338 (August 2021)
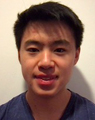
With more than 1.7 million COVID-19 deaths, identifying effective measures to prevent COVID19 is a top priority. We developed a mathematical model to simulate the COVID-19 pandemic with digital contact tracing and testing strategies. The model uses a real-world social network generated from a high-resolution contact data set of 180 students. This model incorporates infectivity variations, test sensitivities, incubation period, and asymptomatic cases. We present a method to extend the weighted temporal social network and present simulations on a network of 5000 students. The purpose of this work is to investigate optimal quarantine rules and testing strategies with digital contact tracing. The results show that the traditional strategy of quarantining direct contacts reduces infections by less than 20% without sufficient testing. Periodic testing every 2 weeks without contact tracing reduces infections by less than 3%. A variety of strategies are discussed including testing second and third degree contacts and the pre-exposure notification system, which acts as a social radar warning users how far they are from COVID-19. The most effective strategy discussed in this work was combined the pre-exposure notification system with testing second and third degree contacts. This strategy reduces infections by 18.3% when 30% of the population uses the app, 45.2% when 50% of the population uses the app, 72.1% when 70% of the population uses the app, and 86.8% when 95% of the population uses the app. When simulating the model on an extended network of 5000 students, the results are similar with the contact tracing app reducing infections by up to 79%.
5) Yunseo Choi (PRIMES) and James Unwin (University of Illinois at Chicago), Racial Impact on Infections and Deaths due to COVID-19 in New York City (11 Jul 2020; arXiv.org , 9 Jul 2020), forthcoming in Harvard Technology Review
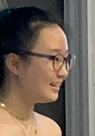
Redlining is the discriminatory practice whereby institutions avoided investment in certain neighborhoods due to their demographics. Here we explore the lasting impacts of redlining on the spread of COVID-19 in New York City (NYC). Using data available through the Home Mortgage Disclosure Act, we construct a redlining index for each NYC census tract via a multi-level logistical model. We compare this redlining index with the COVID-19 statistics for each NYC Zip Code Tabulation Area. Accurate mappings of the pandemic would aid the identification of the most vulnerable areas and permit the most effective allocation of medical resources, while reducing ethnic health disparities.
4) Yi Liang (PRIMES) and James Unwin (University of Illinois at Chicago), COVID-19 Forecasts via Stock Market Indicators (arXiv.org, 13 Dec 2021)
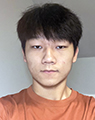
Reliable short term forecasting can provide potentially lifesaving insights into logistical planning, and in particular, into the optimal allocation of resources such as hospital staff and equipment. By reinterpreting COVID-19 daily cases in terms of candlesticks, we are able to apply some of the most popular stock market technical indicators to obtain predictive power over the course of the pandemics. By providing a quantitative assessment of MACD, RSI, and candlestick analyses, we show their statistical significance in making predictions for both stock market data and WHO COVID-19 data. In particular, we show the utility of this novel approach by considering the identification of the beginnings of subsequent waves of the pandemic. Finally, our new methods are used to assess whether current health policies are impacting the growth in new COVID-19 cases.
3) Tanisha Saxena and Daniel Xu, Graph Alignment-Based Protein Comparison (23 Jan 2021)
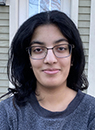
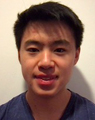
Inspired by the question of identifying mechanisms of viral infection, we are interested in the problem of comparing pairs of proteins, given by amino acid sequences and traces of their 3-dimensional structure. While it is true that the problem of predicting and comparing protein function is one of the most famous unsolved problems in computational biology, we propose a heuristic which poses it as a simple alignment problem, which - after some linear-algebraic pre-processing - is amenable to a dynamic programming solution.
2) Isha Agarwal and Minseo Kim, DP-3T: Ensuring Cryptographic Safety and Security In COVID-19 Contact Tracing , talk presented at the 2021 PRIMES conference
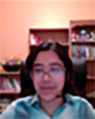
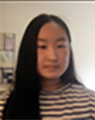
During the COVID-19 pandemic, people have been taking various social as well as innovative measures in order to combat the virus. One of these solutions is contact tracing, which encompasses many different methods, both centralized and decentralized, to identify people who might have been exposed to COVID-19. In our presentation, we deconstruct the mathematics behind the Decentralized Privacy-Preserving Proximity Tracing (DP-3T) algorithm for digital contact tracing and determine some of its potential vulnerabilities. After an analysis of DP-3T, we will provide a brief synopsis of our own proposed improvements, drawing from both pre-existing and novel systems—utilizing RSA encryption and the BB84 quantum computing algorithm—to enhance the cryptographical security of DP-3T.
1) Anish Mudide, SARS-CoV-2 drug discovery based on intrinsically disordered regions , talk presented at the 2020 PRIMES conference
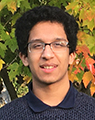
Currently, many drug discovery approaches follow the typical protein structure-function paradigm, designing drugs to bind to fixed three-dimensional structures. However, in recent years such approaches have failed to address drug resistance and limit the set of possible drug targets and candidates. For these reasons we instead focus on targeting protein regions that lack a stable structure, known as intrinsically disordered regions (IDRs). Such regions are essential to numerous biological pathways that contribute to the virulence of various viruses. In our early work, we focus on SARS-CoV-2 therapeutic discovery. Using modern molecular docking procedures and machine learning, we identify eleven new SARS-CoV-2 drug candidates targeting IDR mechanisms. Later, we investigate the use of learned molecular representations for biomimicry-based IDR drug discovery. We find that our model outperforms previous baselines in this area, demonstrating its promise for future work on disordered targets within SARS-CoV-2 or elsewhere.
Contact
With questions, contact PRIMES Program Director Slava Gerovitch at