Philippe Rigollet
Philippe Rigollet is the Cecil and Ida Green Distinguished Professor of Mathematics. His research interests span a wide range of mathematical topics, particularly those emerging from the fields of statistics, data science, and artificial intelligence. Currently, he focuses on statistical optimal transport and the mathematical foundations of Transformers.
Affiliations
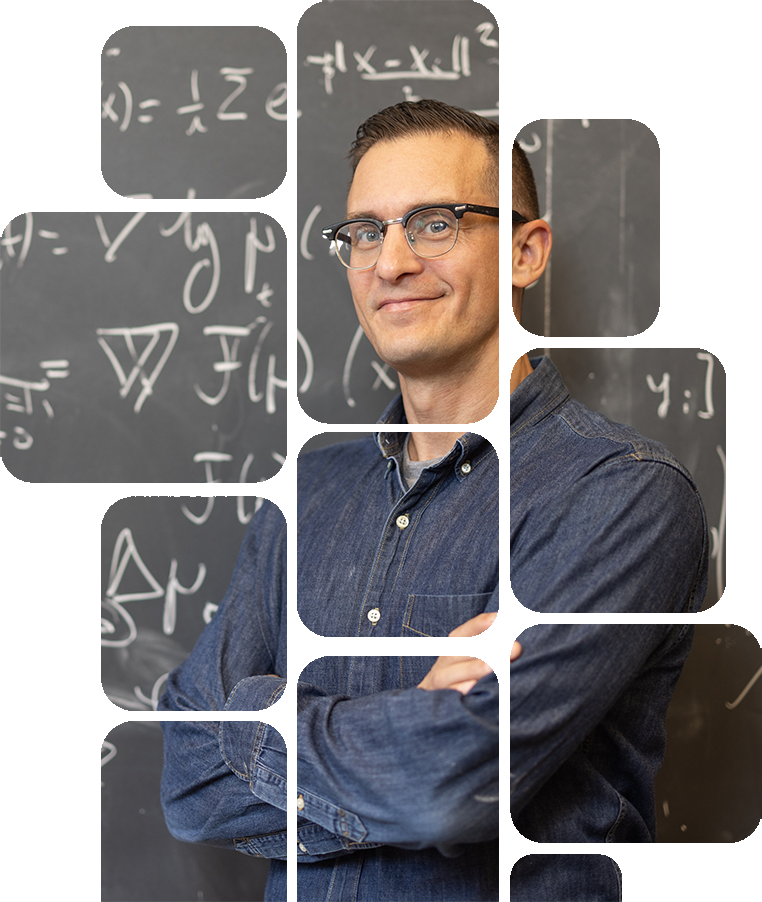
Academic Positions
Professor
MIT, Mathematics, 2020 -
Associate Professor
MIT, Mathematics, 2016 - 20
Assistant Professor
MIT, Mathematics, 2015 - 16
Assistant Professor
Princeton, ORFE, 2008 - 14
Postdoc
Georgia Tech, Mathematics, 2007 - 08
Education & Training
Ph.D. in Mathematics
Univ. of Paris 6 (now Sorbonne Univ.) - 2006
M. Sc. in Statistics & Actuarial Science
ISUP - 2003
B. Sc. in Applied Mathematics
Univ. of Paris 6 (now Sorbonne Univ.) - 2002
Selected Awards
2023
2021
2021
2019
2013
2011
Featured Work
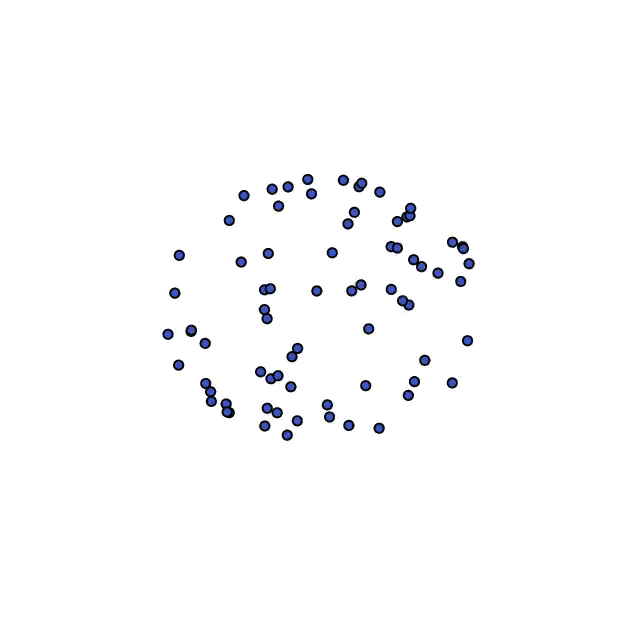
Transformers and Self-Attention dynamics
ArXiv [2305.05465][2312.10794]
Our group recently initiated a line of work where we aim to develop a mathematical perspective on transformers by viewing them as interacting particle systems. As in neuralODEs, we view (self-attention) as velocity fields that evolve particles (token) towards a useful embedding. Our initial work has largely focused on shedding light on the clustering behavior of this system of interacting particles. Even in a very stylized model, many intriguiging mathematical questions arise.
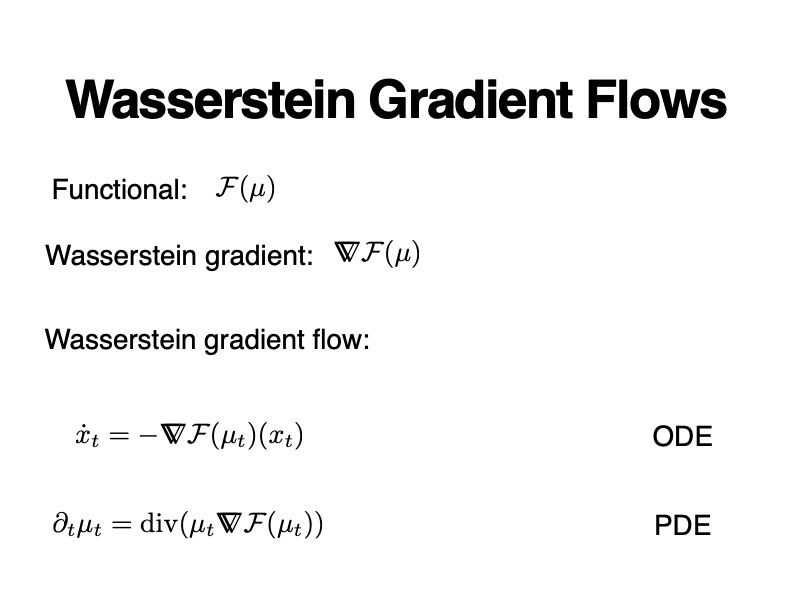
Wasserstein gradient flows
YouTube EBA0NyY4Myc
This talk gives an overview of our recent work on applications of Wasserstein gradient flows to problems arising in statistics and machine learning. The Wasserstein geometry and its extensions (notably Wasserstein-Fisher-Rao) provide a toolbox to develop particle-based optimization algorithms over probability measures. These ideas have been implememented in several examples such as variational inference and nonparametric maximum likelihood estimation.
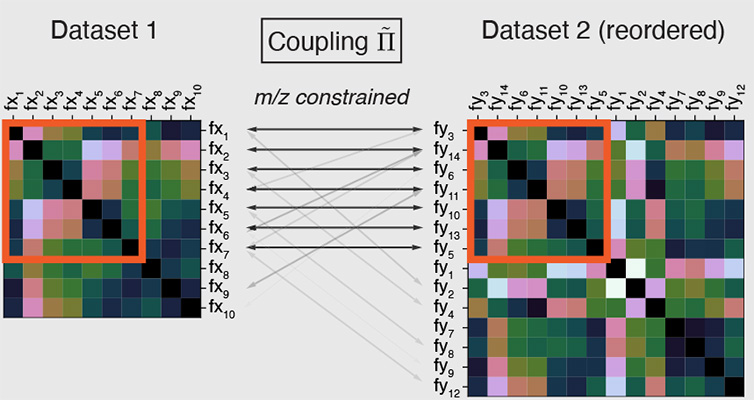
Biological applications
ArXiv 2306.03218
Our group explores applications of novel mathematical ideas to biological data, including genomics data in collaboration with the Eric and Wendy Schmidt Center at the Broad Institute. Our past work has focused on using optimal transport and the Gromov-Wasserstein framework to combine multiple sources of data and we are currently exploring new tools for new applications, including spatial transcriptomics.
Research Group
Openings
Interested in joining our group?
I cannot answer direct requests but you are encouraged to explore the various oppotunites at both the graduate and the postdoc levels. Make sure to check this page regularly, especially in the Fall.
Current Funding
NSF CCF-2106377
Collaborative Research: CIF: Medium: Analysis and Geometry of Neural Dynamical Systems
NSF DMS-2022448
TRIPODS: Foundations of Data Science Institute
Contact
The best way to contact me is via email